AlphaFold
Koza Kurumlu
Science is changing. Previously, scientific discoveries were made purely through the laborious hard work of researchers. However, as humanity steps into the age of technology, computers – more specifically AI models – are aiding us in an unforeseen fashion. Although currently the direct impact of AI in science does not make a noticeable difference, in the next decade it is projected that we will see an exponential growth of its impact on scientific discovery. One of the many projects which have been extremely successful in using AI is AlphaFold, a project poised to revolutionise the world of DNA.
What is AlphaFold?
AlphaFold is an AI model released by DeepMind in 2020 that can predict the structure of a protein given the protein sequence. But what exactly does this mean? Proteins are responsible for nearly every task in cellular life, including cell shape and inner organisation, product manufacture and waste cleanup, and routine maintenance. Moreover, on a bigger scale, proteins help repair and build body tissues, allow metabolic reactions to take place, and coordinate bodily functions. Without proteins, our bodies would not function.
Each protein has a unique structure (the way in which it ‘folds’); in fact, the most important part of understanding proteins is the way it folds. This is because a protein’s structure influences how a it interacts in its environment, defining its function. To understand a protein, knowing its shape is vital.
What Impacts a Protein’s Structure?
A protein is actually a chain of amino acids. These amino acids interact with each other in many ways, such as with hydrogen bonds, hydrophobic interactions, electrostatic interactions and Van der Waals forces. All these interactions affect the folding of the protein. Therefore, if we can model these interactions between the amino acids, we can predict the structure of a protein. This is what AlphaFold has achieved.
Impacts of AlphaFold.
The ability to accurately predict protein structures from their amino-acid sequence, which AlphaFold has provided, is a huge boon to life sciences and medicine. If we know the shape of a protein, we can tell whether it will cause disease or cure a disease. We can tell if it is the protein of a virus, fungi, or harmful bacteria, and we can also find a way to destroy it. For example, the coronavirus vaccine attacks the 3D protein structure on the virus’ spikes. If we know a protein structure, we can even test how the viruses interact with a particular medicine in a computer simulation without ever having to experiment with a human or an animal. This will drastically improve drug discovery and also our understanding of many diseases.
Before AlphaFold, we knew the 3-D structures of only about 17% of the roughly 20,000 proteins in the human body. Those protein structures that we did know had been painstakingly worked out in the laboratory environment over decades, through tedious experimental methods like X-ray crystallography and nuclear magnetic resonance, which require multi-million-dollar equipment and months or even years of trial and error, costing $120,000 to discover the structure of a single protein.
The figure below shows how AlphaFold’s result does not differ much from the experimental results, even though it costs nothing.

[AlphaFold’s prediction overlaid with experimental result]
[https://www.deepmind.com/blog/alphafold-a-solution-to-a-50-year-old-grand-challenge-in-biology]
AlphaFold’s results also crush its competitors by a big margin. AlphaFold’s use of deep neural networks means they don’t actually model each interaction but find a general non-linear mathematical relationship between the chain of amino acids and the way it folds. Since this relationship is trained on thousands of data points, the need to model the interactions (with reinforcement learning) isn’t needed because they are all represented in this ‘general’ formula. Below, we see AlphaFold 2 achieves a median score of 87.0% accuracy.
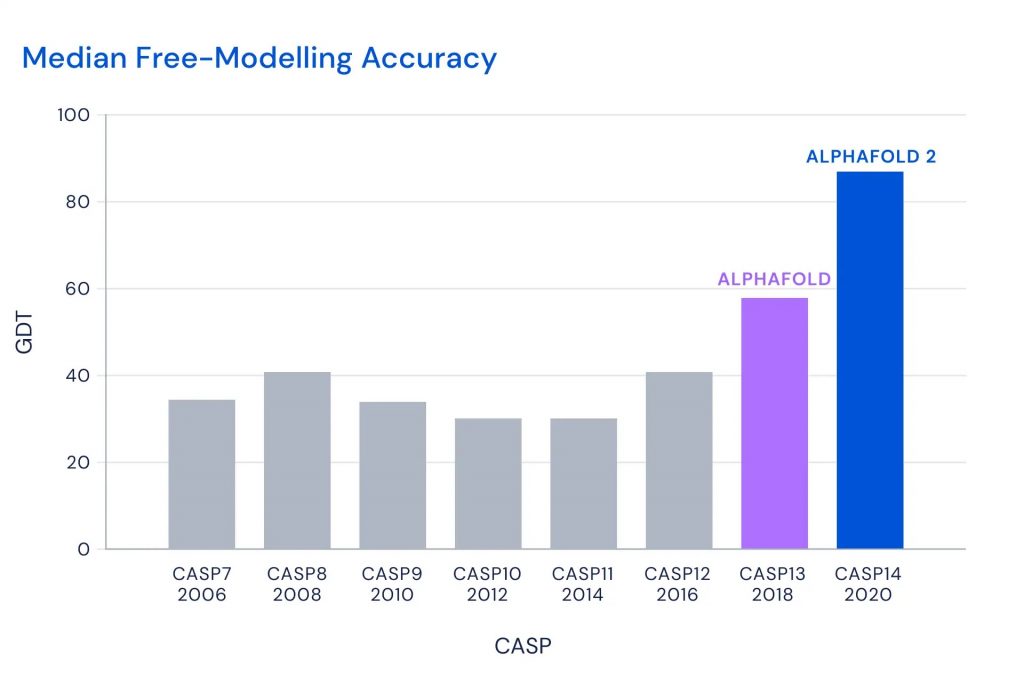
[The increasing modelling accuracy of AlphaFold]
[https://www.deepmind.com/blog/alphafold-a-solution-to-a-50-year-old-grand-challenge-in-biology]
Not only did AlphaFold engineer this model, but they predicted the 3-D structures for virtually all (98.5%) of the human proteome. Of these, 36% are predicted with very high accuracy, and another 22% are predicted with high accuracy. And all of this was released for public use. With the traditional method, this would have cost approximately $43bn, and who knows how many years. The best feature, I argue, of AlphaFold is that it is open-source. Anyone can use AlphaFold’s model, enabling everyone working in medicine or DNA to advance evenly: even beginner researchers. As well as this, it doesn’t need some sort of high-powered expensive computer to run on either; in fact, you could try out the AlphaFold system on your own computer.
Input amino acid sequence:
MAAHKGAEHHHKAAEHHEQAAKHHHAAAEHHEKGEHEQAAHHADTAYAHHKHAEEHAAQAAKHDAEHHAPKP
Output amino acid sequence:
The figure below illustrates the AI model, which essentially is a mathematical relationship which includes variables called parameters that can be slightly altered to affect the results. After an amino acid sequence is passed in and the system has returned a result, the accuracy of the result is calculated by comparing it to the real, experimental result. Next, the parameters are very slightly tweaked to change the accuracy. This is called gradient descent and the ‘tweaks’ are determined by some complex mathematics (partial derivatives of the loss function).
[Figure 3]
There are some limitations of this model, as one might expect. The same protein can sometimes have different forms. AlphaFold currently is not equipped to predict different conformations of the same protein or the outcome of new mutations in a protein’s natural structure due to viruses. Nonetheless, besides AlphaFold’s tremendous capabilities and added value to biology, it also marks an important benchmark in which AI is beginning to take over scientific research.
References:
https://www.deepmind.com/blog/alphafold-a-solution-to-a-50-year-old-grand-challenge-in-biology